The Development of AI-Driven E-Discovery Tools
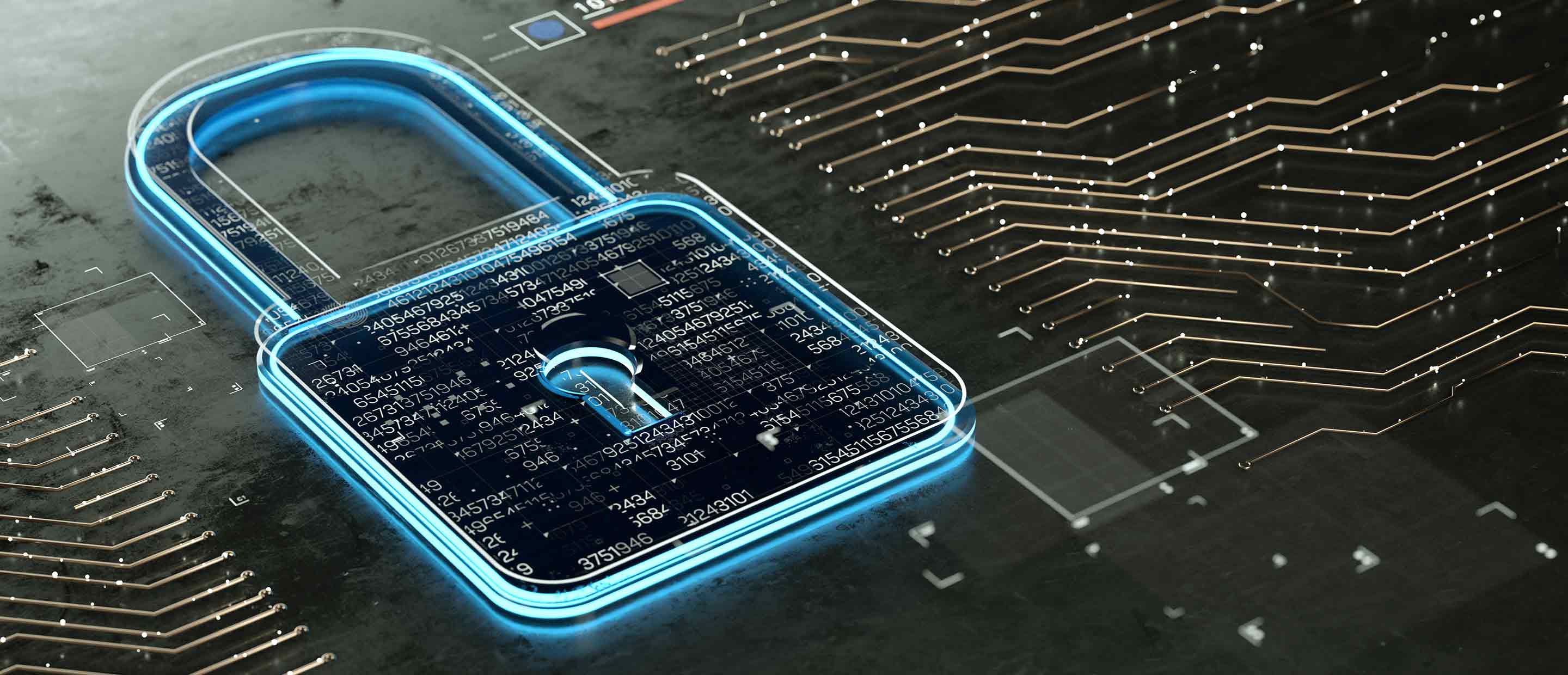
Recent headlines have been dominated by rapid developments in generative artificial intelligence, and a number of startups are positioning themselves to offer new tools to the legal industry making use of this groundbreaking technology. While these developments may change legal practice going forward, machine learning and other powerful algorithms already play a huge role in modern lawyering. It wasn’t long ago that “electronic discovery” was a nascent and cutting-edge concept. But most of today’s litigators have now firmly exchanged banker’s boxes and microfiche for search terms and technology-assisted review. This article looks at how many technologies frequently described as AI have already reshaped litigation practice.
The Shift to eDiscovery: A Problem of Volume
“AI” is a catchall term that can mean many things, and new generative AI technologies have the potential to transform legal research and drafting. But machine learning algorithms have already made their mark on legal discovery as a means to streamline document review. The shift to electronic discovery has relieved some burdens of discovery, as attorneys no longer need to sift through mountains of paper documents, but that shift has also added new challenges due to the sheer volume of electronic communication that underlies most business activity. Document review is thus often the most burdensome and expensive single part of the discovery process.
Ever since the transition to primarily electronic discovery, law firms and specialized vendors have been looking for ways to make review more efficient. Relativity, which offers of a common document review database platform, launched an analytics product in 2008, and offered its first technology-assisted review (TAR) product in 2012. TAR algorithms help lawyers identify relevant documents in a litigation. In a typical TAR process, attorneys will code a randomly generated “seed set” of documents, which are then used to train the model. Once the model has been trained, it can either be used to rank documents on likely responsiveness or even apply predictive coding directly.
Early Judicial Acceptance of Technology-Assisted Review
The use of predictive coding in document reviews was first accepted by a federal court that same year when Magistrate Judge Andrew Peck of the Southern District of New York explicitly blessed the practice in Da Silva Moore v. Publicis Groupe. 287 F.R.D. 182, 193 (S.D.N.Y. 2012). Judge Peck put the bar on notice that “computer-assisted review is an available tool and should be seriously considered for use in large-data-volume cases where it may save the producing party (or both parties) significant amounts of legal fees in document review.” 287 F.R.D. 182, 193 (S.D.N.Y. 2012). In recognizing the value of TAR in reducing litigation costs, the court in Da Silva Moore also noted that there were risks posed by relying on AI to make document production decisions, and noted that agreement about the use of TAR in the first instance, and transparency of the process used, were important considerations.
Further Developments in Technology and the Law of AI Discovery
At issue in Da Silva Moore was what some eDiscovery experts call TAR 1.0, which is characterized by the use of Simple Active Learning or Simple Passive Learning algorithms. These TAR 1.0 algorithms relied on a single static training set to train an algorithm, which would then apply predictive coding to an entire review set based on that training set.
These early models have largely been replaced by a new process, sometimes called TAR 2.0, which relies on Continuous Active Learning (CAL) models. Like the TAR 1.0 algorithms, a CAL model is initially trained on a seed set of documents reviewed by subject matter experts, but unlike those earlier algorithms, a CAL model will then rank documents based on likelihood of responsiveness. Human reviewers will then conduct a prioritized review based on the ranking generated by the model, which will continuously update itself based on the responsiveness tagging completed by those human reviewers.
As the technology has evolved and the use of TAR in document reviews has become commonplace in data- and document-heavy cases, negotiations regarding requests for production now regularly focus on TAR parameters in addition to document custodians, relevant time periods, and search terms. Like all discovery issues, the specifics of TAR parameters have been litigated in a variety of contexts including when use of TAR is appropriate, what TAR parameters are permissible in a given context, and how much information about its TAR process a party must share with its opponent. The Sedona Conference recently published the second edition of its helpful primer on TAR case law. The breadth of issues addressed in the case law highlights how commonplace this version of AI has become in legal practice, and it is now “‘black letter law’ that courts will permit a producing party to utilize TAR.” Entrata, Inc. v. Yardi Sys., Inc., No. 2:15-cv-00102, 2018 WL 5470454, at*7 (D. Utah Oct. 29, 2018).
That is not to say that there are not concerns that need to be addressed as the e-discovery field adopts new forms of AI-driven document review technologies. Concerns of AI “hallucinations” are more easily managed in e-discovery than in other AI applications because of the closed universe of searchable documents. However, that does not mean that using AI models will be appropriate for all e-discovery projects or that attorneys can abdicate their oversight over document discovery to AI. Judge Peck’s words from Da Silva in 2012 apply equally well today: while “computer-assisted review is not a magic, Staples-Easy-Button, solution appropriate for all cases,” instead “[t]he goal is for the review method to result in higher recall and higher precision than another review method, at a cost proportionate to the ‘value’ of the case.”1 The risk tied to these improvements must be managed as it is currently, through negotiation and restriction setting with the opposing party and oversight with human review and verification.
A Well-Developed Field
Use of TAR is now widespread, and practitioners have guidance in how to use it. For example, the Bolch Judicial Institute at Duke Law school has published a helpful set of TAR Guidelines to guide practitioners through the nuances of TAR. Lawyers and discovery professionals at Vinson & Elkins are experienced in using TAR to keep discovery costs down for clients.
Some predict that new developments in AI will further transform the discovery process by bringing more sophisticated models like those used in ChatGPT to bear on the document review process. But unlike in other facets of the law, like research and drafting, where the use of AI will work a sea change, new applications of AI in discovery will be more like refinements to a well-established system. Like all elements of discovery, issues arising out of the use of AI technology will surely be well litigated, but the judges, magistrate judges, and even special masters appointed to oversee discovery issues are at this point well-accustomed to resolve these sorts of disputes.
1Da Silva Moore v. Publicis Groupe, 287 F.R.D. 182 (S.D.N.Y. 2012).
Related Insights
- Event RecapMay 16, 2024Video
- Insight
Published by ESG Investor February 16, 2024
April 2, 2024 - InsightApril 2, 2024
This information is provided by Vinson & Elkins LLP for educational and informational purposes only and is not intended, nor should it be construed, as legal advice.